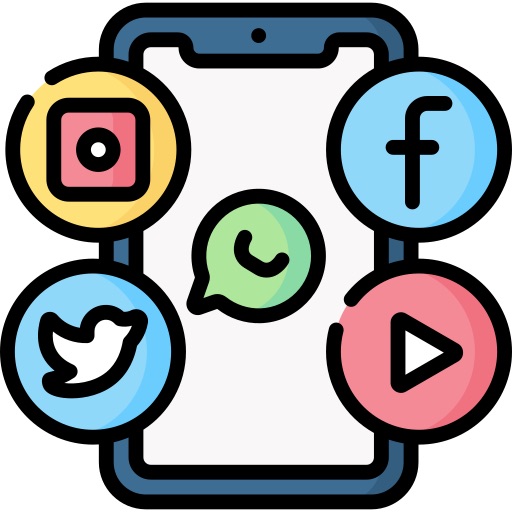
Towards Identifying, Understanding and Controlling Cumulative Revelations in Social Media.
Emma Nicol, Amal Htait, Leif Azzopardi, and Wendy Moncur.
Association for Information Science and Technology (ASIS&T). 2021.
☆ Describes some findings from interviews about people’s online interactions, specifically focusing on their requirements and desires for improved ways to identify, understand and control the cumulative revelations that may arise from their social media profiles, and the pieces of information that, when put together, could put them at risk of grave consequences.
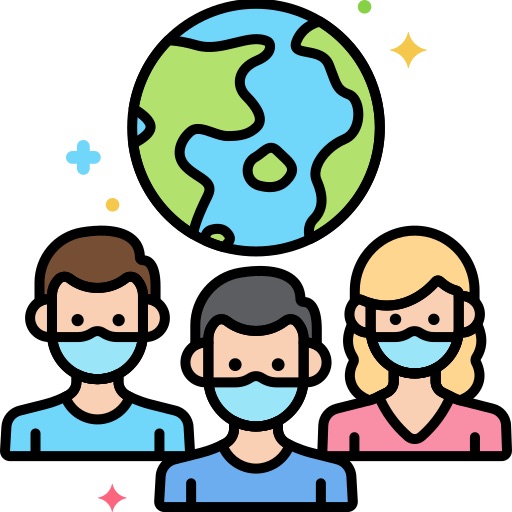
Data, socially distanced: cumulative data disclosure and data narratives during Covid.
Burkhard Schafer, Emma Nicol, Wendy Moncur, Amal Htait, Joe Briggs, Leif Azzopardi, and Daniel Carey.
British and Irish Law Education Technology Association Conference (BILETA). 2021.
☆ Discusses methodological issues of a research project into legal and technological responses to the risk of cumulative disclosure of data. We conducted qualitative interviews with 26 participants, which examined their personal digital ecosystems. Crucially, the beginning of the study coincided with the outbreak of the COVID pandemic, which meant that the interviewees were experiencing an unprecedented need to adjust their own online presence and working patterns.
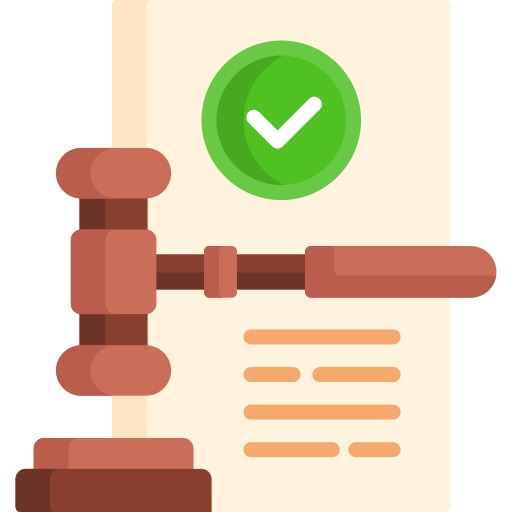
Death by a Thousand Cuts: Cumulative Data Effects and the Corbyn Affair.
Burkhard Schafer.
Datenschutz und Datensicherheit-DuD. 2021.
☆ Analyses some of the legal issues behind the Corbyn affair and focusses in particular on an emerging conflict between equality legislation and data protection law, a conflict that is rooted in diverging approaches to cumulative versus atomistic online harms and their impact on human dignity, free speech and equality.
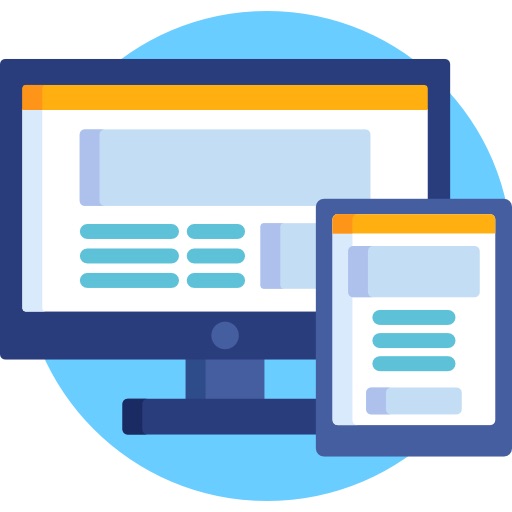
Four Speculative Design F(r)ictions: Designing for Personal Data Awareness.
Daniel Carey, Callum Nash, Luke Sellers and Jo Briggs.
Human-Data Interaction through Design Workshop: CHI2021. 2021.
☆ Presents four speculative design f(r)ictions as critical framing devices to help people engage with, reflect upon, and understand obfuscated personal data processes.
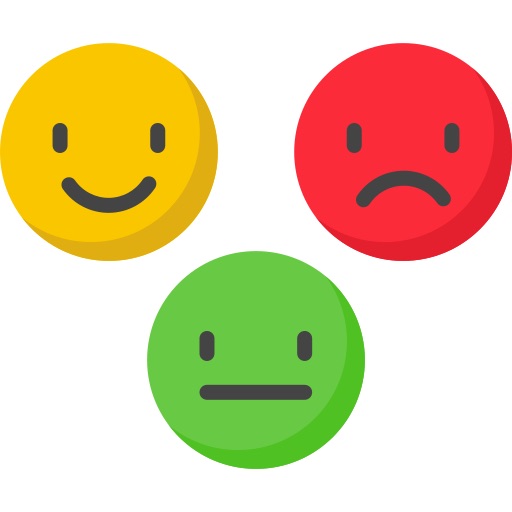
AWESSOME: An Unsupervised Sentiment Intensity Scoring Frameworkusing Neural Word Embeddings.
Amal Htait and Leif Azzopardi.
The 43rd European Conference on Research and Development in Information Retrieval (ECIR). 2021.
☆ Presents a sentiment intensity scoring framework that generalizes and combines past works of pre-existing lexicons (e.g. VADER) and neural word embeddings (e.g. BERT) to address the sentiment intesity scoring problem, with no required training, and while providing fine grained SIS of words and phrases.
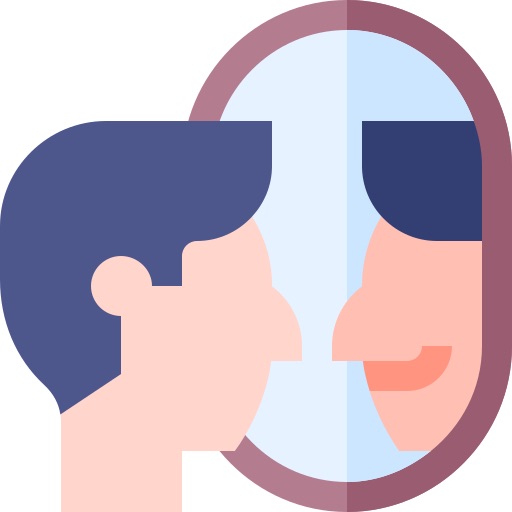
DataMirror - Reflecting on One's Data Self: A Tool for Social Media Users to Explore Their Digital Footprint.
Amal Htait, Leif Azzopardi, Emma Nicol, and Wendy Moncur.
The 43rd International ACM SIGIR Conference on Information Retrieval (SIGIR). 2020.
☆ Presents an initial prototype tool, that enables social network users to aggregate their online data so that they can search, browse and visualise what they have put online.
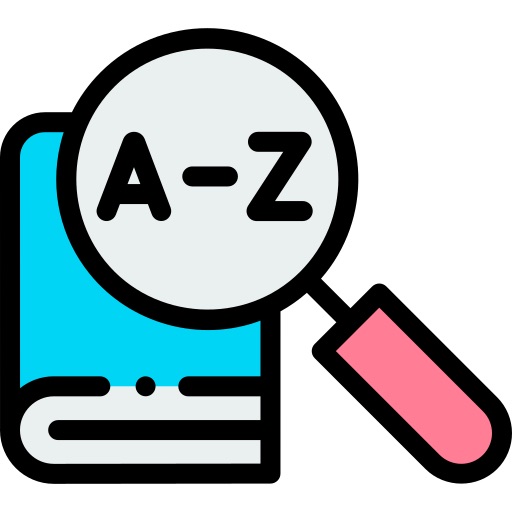
Using Sentiment Analysis for Pseudo-Relevance Feedback in Social Book Search.
Amal Htait, Sébastien Fournier, Patrice Bellot, Leif Azzopardi, and Gabriella Pasi.
The ACM SIGIR International Conference on the Theory of Information Retrieval (ICTIR). 2020.
☆ Presents a sentiment-oriented method for the selection of sentences from the reviews of top rated book. From these sentences, we extract the terms to be employed in the query reformulation. The sentence selection process is based on a semi-supervised SA method, which makes use of adapted word embeddings and lexicon seed-words.
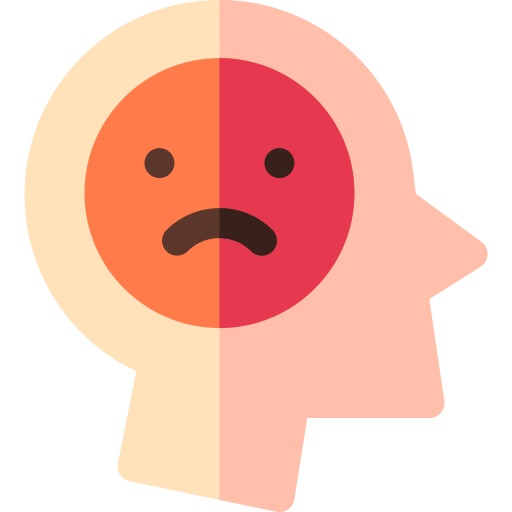
Early Risk Detection of Self-Harm and Depression Severity using BERT-based Transformers.
Rodrigo Martínez-Castaño, Amal Htait, Leif Azzopardi, and Yashar Moshfeghi.
The 11th edition of Conference and Labs of the Evaluation Forum (CLEF) - eRisk Working Notes. 2020.
☆ Presents a brief description of our research groups' efforts in tackling Task 1 (Early Detection of Signs of Self-Harm), and Task 2 (Measuring the Severity of the Signs of Depression) from the CLEF eRisk Track. Core to how we approached these problems was the use of BERT-based classifiers which were trained specifically for each task.